How AI is accelerating the Energy Transition and carbon negative
Author Sticky
Nations across the globe and the energy and industrial sectors are experiencing the impact of the energy transition, as governments and corporations progress toward reaching a low-carbon footprint. The impact varies depending on net zero goals, investment, infrastructure, and workforce readiness.
But more than reaching net zero goals, the real end game is to achieve carbon negative, when you remove more carbon dioxide and carbon dioxide equivalent (CO2e) greenhouse gasses than you emit. It will happen in phases, and some countries and industries will reach goals faster, but one point is unanimous among industry experts: without Artificial intelligence (AI) there is no energy transition and no carbon reduction.
According to a BloombergNEF (BNEF) report published by the World Economic Forum, AI has the potential to create substantial value for the global energy transition. Based on BNEF’s net zero scenario modelling, every 1% of additional efficiency in demand creates $1.3 trillion in value between 2020 and 2050 due to reduced investment needs. Using AI in the energy sector could achieve this by enabling greater energy efficiency and flexing demand.
So it’s no surprise the Global Artificial Intelligence Industry Forecast indicates that the global AI market was valued at around $62.35 billion in 2020, and it is expected to grow at a compound annual growth rate (CAGR) of nearly 40% during the forecast period from 2021 to 2026.
But more than reaching net zero goals, the real end game is to achieve carbon negative, when you remove more carbon dioxide and carbon dioxide equivalent (CO2e) greenhouse gasses than you emit. It will happen in phases, and some countries and industries will reach goals faster, but one point is unanimous among industry experts: without Artificial intelligence (AI) there is no energy transition and no carbon reduction.
According to a BloombergNEF (BNEF) report published by the World Economic Forum, AI has the potential to create substantial value for the global energy transition. Based on BNEF’s net zero scenario modelling, every 1% of additional efficiency in demand creates $1.3 trillion in value between 2020 and 2050 due to reduced investment needs. Using AI in the energy sector could achieve this by enabling greater energy efficiency and flexing demand.
So it’s no surprise the Global Artificial Intelligence Industry Forecast indicates that the global AI market was valued at around $62.35 billion in 2020, and it is expected to grow at a compound annual growth rate (CAGR) of nearly 40% during the forecast period from 2021 to 2026.
Defining AI in Energy and Other Heavy Industries
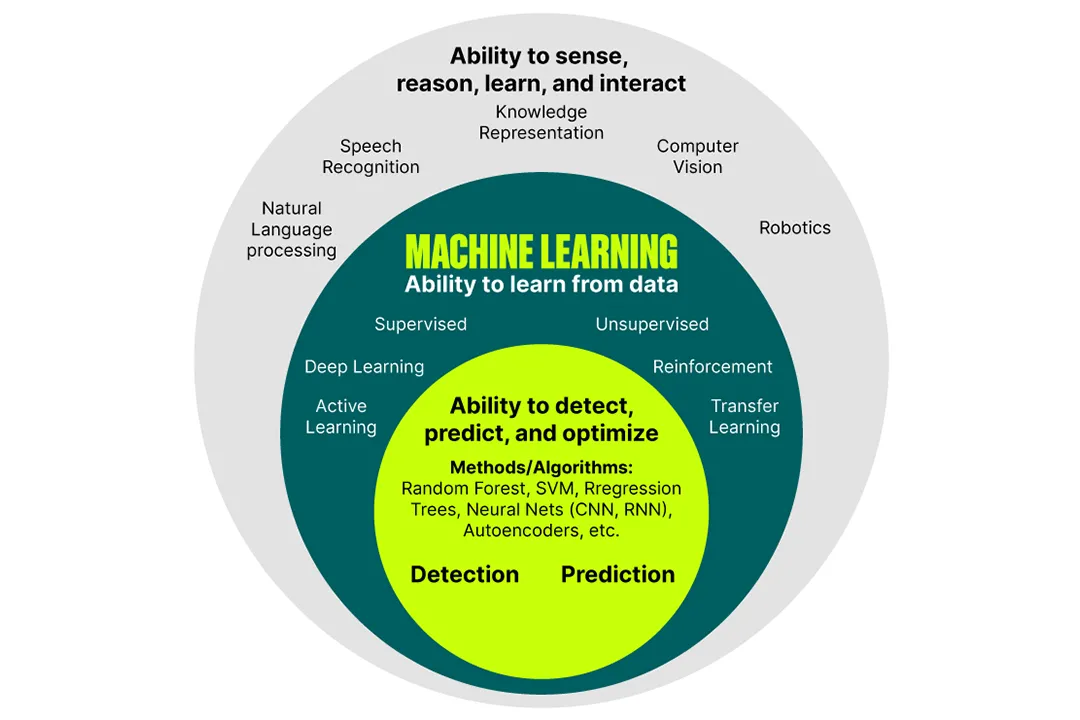
At GE Vernova, the definition of Artificial Intelligence represents the ability to 1) Sense, 2) Reason, 3) Learn, and 4) React with human-like levels of intelligence. Machine learning is a subset of AI involving the use of algorithms to learn from data and improve performance on a specific task without being explicitly programmed.
After decades of collecting information, companies in the energy industry, such as power generation, oil and gas, chemicals, metal and mining, are often data rich but insights poor, making it almost impossible to navigate the millions of records of structured and unstructured data to find relevant information. This challenge is particularly important when engineers are troubleshooting new issues on highly complex systems to find the most relevant procedures, machine performance data, history of operations, and instances of relevant issues on similar subsystems. Engineers are often left relying on their previous experience, talking to other experts, and searching through piles of data to find relevant information. For critical issues, this high-stakes scavenger hunt is stressful at best and often leads to suboptimal outcomes, said McKinsey & Company in its report on the promise of AI in industrials.
In a recent study conducted by Verdantix on industrial AI analytics, researchers noted that the early days of advanced AI analytics saw improvements in training techniques, the proliferation of inexpensive data acquisition, cloud-scale big data acceleration and the arrival of purpose-built computer hardware to speed up development. Building on these established workflows, industrial firms are investing in and seeing success with several core AI technologies including the following:
After decades of collecting information, companies in the energy industry, such as power generation, oil and gas, chemicals, metal and mining, are often data rich but insights poor, making it almost impossible to navigate the millions of records of structured and unstructured data to find relevant information. This challenge is particularly important when engineers are troubleshooting new issues on highly complex systems to find the most relevant procedures, machine performance data, history of operations, and instances of relevant issues on similar subsystems. Engineers are often left relying on their previous experience, talking to other experts, and searching through piles of data to find relevant information. For critical issues, this high-stakes scavenger hunt is stressful at best and often leads to suboptimal outcomes, said McKinsey & Company in its report on the promise of AI in industrials.
In a recent study conducted by Verdantix on industrial AI analytics, researchers noted that the early days of advanced AI analytics saw improvements in training techniques, the proliferation of inexpensive data acquisition, cloud-scale big data acceleration and the arrival of purpose-built computer hardware to speed up development. Building on these established workflows, industrial firms are investing in and seeing success with several core AI technologies including the following:
- Anomaly detection to identify irregularities.
- Computer vision to monitor assets and processes through images and video.
- AI forecasting to support predictive analytics.
- Natural language processing to improve data discoverability.
In this same study, Verdantix compared GE Vernova with several other vendors showing which vendors have maximized the use of AI in seven use cases to help organizations to accelerate through the energy transition. GE Vernova was one of three vendors to offer software in each category:
- Asset Information Management
- Asset Condition Monitoring
- Predictive Maintenance
- Production and Process Management
- Supply Chain Optimization
- Energy Management
- ESG Tracking and Reporting
Use Cases of AI for Predictive Maintenance
Asset-intensive industries need to maintain critical assets and equipment free of failures and unplanned maintenance, otherwise it can negatively impact operations and quickly bring millions of dollars of cost.
By using predictive analytics enabled by digital twin blueprints, Asset Performance Management (APM) software provides early detection of pending issues, diagnostic analytics and indicates probable cause, suggesting maintenance alternatives. Deploying APM for customers, GE Industrial Managed Services has demonstrated $1.6B in Operations & Maintenance (O&M) savings.
Let’s break down the AI embedded in APM. Digital Twins are build based on OEM expertise and then trained to be more accurate and high performing with AI/ML. AI for Digital Twins allows for cloud customers to get time to action projections that support the predictive element of the use case. AI/ML then takes the data from catches and uses them in future decisions—which is the intelligence piece of the software. More data = more accurate projections.
By using predictive analytics enabled by digital twin blueprints, Asset Performance Management (APM) software provides early detection of pending issues, diagnostic analytics and indicates probable cause, suggesting maintenance alternatives. Deploying APM for customers, GE Industrial Managed Services has demonstrated $1.6B in Operations & Maintenance (O&M) savings.
Let’s break down the AI embedded in APM. Digital Twins are build based on OEM expertise and then trained to be more accurate and high performing with AI/ML. AI for Digital Twins allows for cloud customers to get time to action projections that support the predictive element of the use case. AI/ML then takes the data from catches and uses them in future decisions—which is the intelligence piece of the software. More data = more accurate projections.
Gaining Insights into Future Capacity and Energy Prices
Energy market data is more diverse and decentralized than ever, especially now with the addition of renewable energy into the mix. An overwhelming amount of information bombards energy traders daily in today’s market, with the sheer volume and complexity making uniting and analyzing the information quickly enough to anticipate market movements in time to capture value challenging.
GE Vernova has created a unique solution that leverages advanced AI to produce generation and price predictions, then combines them with a risk management approach matched to the customer’s risk profile.
GE Vernova has created a unique solution that leverages advanced AI to produce generation and price predictions, then combines them with a risk management approach matched to the customer’s risk profile.
AI Transforming Sorgenia’s Approach to Maintenance
Leading Italian digital energy company Sorgenia uses GE Vernova’s APM Reliability software deployed in the cloud to maximize the reliability and efficiency of its state-of-the-art, combined-cycle gas turbine power plants. According to the company, the software has given them insights to better plan maintenance around annual outages, specifically at their Termoli and Modugno plants. Doing so allows them to avoid unnecessary downtime on critical assets, such as heat recover steam generators or condensers, which in turn allows them to operate at higher efficiency and satisfy their more than 450,000 customers’ need for electricity. Read more in the 2022 GE Sustainability Report.
AI Helping to Reduce Emissions and Fuel Consumption
Manual tuning of gas turbines traditionally occurs twice a year during seasonal transitions. However, weather is unpredictable, and fuel is a major expense for thermal generators. With the integration of AI/ML, GE Vernova’s Autonomous Tuning enables power generators worldwide in achieving automated gas turbine tuning to optimize for all current conditions and transitional modes. The gas turbine performance software solution allows for finding and maintaining the turbines optimal operating conditions, referred to as the ‘best zone’ or ‘sweet spot’. The AI/ML algorithms continuously analyze real-time data to adopt and optimize to variable changes, such as shifts in environmental conditions, variations in fuel properties, and physical degradation.
As a result, power generation plants have achieved impressive environmental and efficiency improvements, including 14% reduction in carbon monoxide emissions, 10% to 14% decrease in nitrous oxide emissions, and noteworthy reductions in fuel consumptions and CO2 emissions, ranging between .5-1%.
“With Autonomous Tuning, GE Digital has introduced a practical industrial example of the use of machine learning in closed loop supervisory control, and all running at the Edge,” according to Joe Perino, Principal Analyst at LNS Research. “This is a real-world application of AI for decarbonization with tangible reductions in emissions and fuel for gas turbine operators.
As a result, power generation plants have achieved impressive environmental and efficiency improvements, including 14% reduction in carbon monoxide emissions, 10% to 14% decrease in nitrous oxide emissions, and noteworthy reductions in fuel consumptions and CO2 emissions, ranging between .5-1%.
“With Autonomous Tuning, GE Digital has introduced a practical industrial example of the use of machine learning in closed loop supervisory control, and all running at the Edge,” according to Joe Perino, Principal Analyst at LNS Research. “This is a real-world application of AI for decarbonization with tangible reductions in emissions and fuel for gas turbine operators.
From Manual to Digital Inspections
GE Vernova is collaborating with large power generation organizations by providing a cloud-based image analytics application that automates the manual data collection to insight generation process. It enables seamless collection and transfer of image data to cloud which is then processed by AI-enabled algorithms to generate valuable insights and triggers actionable alerts. Thus, it empowers customers to make informed decisions fast and take proactive measures to optimize their asset and operational performance. Autonomous imaged-based inspections can save a significant amount of cost. Research shows approximately 20% cost savings on labor and asset maintenance.
AI-Empowered Carbon Emissions Management Software
Effective emissions management must begin with accurate measurement of Greenhouse Gas Emissions (GHG). Not only to align with stricter emissions reporting mandates, but to enable progress on carbon negative goals. Data must be combined with analysis of what methods would be effective to reduce emissions and what the impact would be on the organization.
GE Vernova’s recently launched CERius™ Emissions Management Software automates more accurate GHG data collection and reporting, performs predictive analysis including what-if scenarios, and recommends actions to reduce them. The software eliminates manual data collection and siloes between sites and departments and effectively ties emissions data to decarbonization strategy.
In summary, AI can be a powerful tool in the fight against climate change and in achieving carbon negativity. By applying energy transition technologies built with AI/ML strategically in various sectors, we can reduce carbon emissions and increase the capacity to remove carbon from the atmosphere, ultimately helping to address the global climate crisis.
GE Vernova’s recently launched CERius™ Emissions Management Software automates more accurate GHG data collection and reporting, performs predictive analysis including what-if scenarios, and recommends actions to reduce them. The software eliminates manual data collection and siloes between sites and departments and effectively ties emissions data to decarbonization strategy.
In summary, AI can be a powerful tool in the fight against climate change and in achieving carbon negativity. By applying energy transition technologies built with AI/ML strategically in various sectors, we can reduce carbon emissions and increase the capacity to remove carbon from the atmosphere, ultimately helping to address the global climate crisis.